Dr Stephanie Brooks, Foods Connected’s Head of Innovation, breaks down what businesses need to know about AI and how to implement it into the agri-food sector.
When we talk about artificial intelligence we think of computers performing tasks, tasks that historically required human intelligence. Systems set to work recognising speech, making decisions and identifying patterns.
But AI is expanding and today encompasses a wide variety of technologies. Machine learning (ML) sees algorithms trained on data sets to create models that allow computer systems to perform tasks like making recommendations, identifying optimal travel routes (google maps predicting traffic delays), and translating text between languages. With deep learning, networks with multiple layers are being used to learn complex patterns from data, while computers are being taught to understand, interpret, and generate human language in natural language processing (NLP).
But there are some systemic challenges around AI in agri-food. These include:
- Organisational readiness and willingness to adopt
- Financial investment and the need for a clear ROI
- And a skilled workforce including data scientists and AI specialists.
The two areas of AI
As AI becomes more advanced two different areas are emerging – traditional and generative AI. The first – also known weak AI or narrow AI - focuses on specific tasks using predefined rules. Examples of this include voice assistants like Siri, recommendation engines on Netflix and Google’s search algorithm. We think of them as smart rule-followers because while they follow specific instructions, they don’t create anything new.
There are many existing applications for this currently in agri-food. Here are some examples:
- Vision and ML systems such as video image analysis (VIA) in meat grading, behavioural analysis in livestock
- ML applications in testing e.g. Bia Analytical
- Preventative maintenance e.g. John Deere has ML embedded in its agri equipment
- Vision systems in factories for quality inspections and defect detection
Then we have Generative AI, which can learn, adapt and generates fresh ideas, leveraging machine learning techniques to create new content. ChatGPT and other Large Language are primary examples. This is currently more novel in our sector.
So, what do agri-food businesses need to do to be ready when it comes to implementing AI? As I see it there are five basic ingredients that need to be in place - organisational readiness, digital data collection, infrastructure, data readiness and the necessary skills to make the rest work.
The first steps towards implementation
The first step is to practically manage this implementation across agri-food through a combination of digitalisation and data readiness. It is about transformation and a conversion of analogue data into a digital format, This involves integrating digital technologies into business processes, to improve efficiency and enable new forms of value creation. In addition, it is about ensuring data is "ready"- data that is prepared, accessible and suitable for analysis and decision making so that it can be used as a strategic asset.
"The first step is to practically manage this implementation across agri-food through a combination of digitalisation and data readiness."
It will take work – there is no such thing as “simply digitising”. It will take three factors – provision, people and practicalities – all working together then it’s down to building confidence in the sector that adoption is the way forward. Let's look at why these factors are so important to the process.
Provision
It’s not that technologies haven’t been developed and tested – they have. It’s that less attention has been paid to actually provisioning tech specifically for the agri-food industry. Often technologies that make their way into the agri-food sector have been developed with a use case or other industry in mind. Only after this is the potential for it to be applied within agri-food production and/or manufacturing recognised.
Look at blockchain – its origins are in fintech, designed to trade digital currency bitcoin without the need for a trusted authority such as a bank. But repurpose this technology into the agri-food space, where it was pivoted to be an emerging technology to disincentivise and prevent fraud, and it’s clear it isn’t completely fit for purpose in its legacy form.
Why? Because the decentralised nature of blockchain means that using a typical payment model based on the number of transactions, where there are many transactions, is just not possible in a high volume/low margin sector like agri-food. So, in reality it, is just too costly to roll out. An obvious takeaway here is that if technologies are to be adapted and provisioned with agri-food in mind, then it is vital that the technology providers have an in-depth knowledge of the agri-food sector.
People
And it isn’t just provisioning where technology adoption currently falters. We need to shine a spotlight on how we get people to buy in. The breakdown here might not be where you think. While the benefit technology can bring to businesses is often understood on a macro level within the food industry, particularly by those in thought leadership positions, it is the onboarding of this technology’s main users that has proved more difficult.
"If technologies are to be adapted and provisioned with agri-food in mind, then it is vital that the technology providers have an in-depth knowledge of the agri-food sector."
This is not generally due to the people, but because of the change it involves. The benefits of this new way of working are not always immediately or overtly obvious to users. In fact, as a technology provider, I am often met with statements of resistance, such as: “because this is the way it has always been done”. However, this struggle to accept new technologies is often associated with a fear of change and being replaced by machines. That’s why it is so important that technology providers acknowledge these concerns and take them into consideration, reassuring users of the benefits during implementation.
Practicality
Addressing the practical aspects of a digital way of working within agri-food is the final part of making technology adoption more accessible. The responsibility here lies with the tech providers.
The processes, changes, and practical steps required to implement a new tech into a business are often confused and even misunderstood, even by the technology companies themselves.
In general, the agri-food sector operates on a high-volume low-margin model, meaning that processes are tightly refined and controlled. The industry has worked hard over the last 20+ years to bring about operational efficiencies through automation, so that cost savings or profitability can be achieved.
During this period there has been less of a focus on digitising data and the value it could bring through actionable insights. When disruptive technologies enter this process, they need to do so with ease and not impact operations or processes. In addition, they need to add value rather than create costs for businesses.
Furthermore, these technologies need to be able to cope with the intricacies and nuances that exist in the production and manufacturing environments. Put simply, sometimes technology will not be plug and play – especially in its infancy.
"Put simply, sometimes technology will not be plug and play – especially in its infancy. "
The outcome here is that the inherent benefits of digitisation are not immediately obvious, as they would be with operation efficiency gains. It is critical that technology providers take the time to understand the specific business where they are attempting to implement technologies and provide solutions that will support and enhance the business.
What is clear is that data quality, availability and control are key and that food businesses need to move their information into a data lake now – even if it isn’t organised (yet) in the best possible way. This will ensure that when you do have the wider digital processes and procedures in place that you’ll also have the historical data to draw upon to give your analysis context.
So what can businesses be doing now?
Start by digitising your data to enable efficient collection, analysis and interpretation. Make sure you use high quality, accessible data in a standardised format to allow data exchange,as you will need to integrate it from various sources and formats. And use real time or near real time from multiple sources to ensure timely decision making
Then work through these five steps:
- Digitalisation
- Develop AI use cases specific to your business
- Understand your data readiness position
- Extract, transform and load currently digitally available data into a lake/warehouse
- Adopt a scalability strategy, scaling technologies and implementing master data control with AI in mind
Our in-house AI project
At Foods Connected we are working across a number of AI deliverables. We are building a PoC generative AI chatbot (think ChatGPT) housed within Foods Connected that customers can ask questions and receive answers back instantly. An example would be “What raw materials are sourced from country x?” We are also developing a rapid test (with ML/AI models) that can be utilised within industry settings to determine origin of soya. As well as this we have established an AI Governance Group to ensure responsible implementation in this project and beyond.
I’ll be speaking more on this subject at Proactive Food Safety Conference in Amsterdam next week.
If you’d like to know more about our AI projects or learn about we can help with your digital transformation then request a demo today, or contact us to discover how we can keep your food business agile in an ever-changing market.
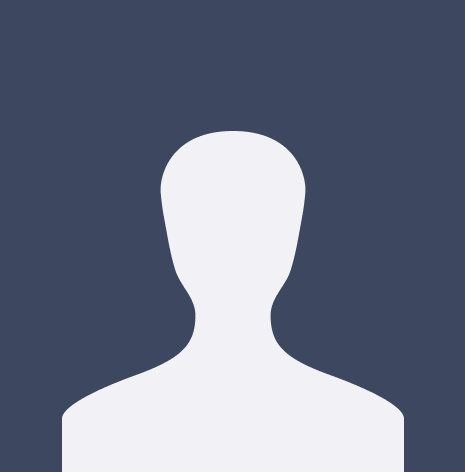
Dr Stephanie Brooks
Stay up to date
Stay up to date
Browse Posts
- May 2025
- April 2025
- March 2025
- February 2025
- January 2025
- December 2024
- November 2024
- October 2024
- September 2024
- August 2024
- July 2024
- June 2024
- May 2024
- April 2024
- March 2024
- February 2024
- January 2024
- December 2023
- November 2023
- October 2023
- September 2023
- August 2023
- July 2023
- June 2023
- May 2023
- April 2023
- March 2023
- December 2022
- November 2022
- October 2022
- September 2022
- August 2022
- July 2022
- June 2022
- May 2022
- April 2022
- March 2022
- February 2022
- January 2022
- December 2021
- November 2021
- October 2021
- August 2021
- July 2021
- June 2021